In the past I have ranted about the evils of p-values and also how we’re not collecting the right sort of data. Both of these have just collided in my work, and I’m not sure what to do.
The problem (simplified, and with details removed to protect the guilty) is this. We have a large microarray study. The data are expression levels of thousands of genes in some treatments, with a few random effects thrown in as well. What we want to do it to pick out the interesting genes, i.e. those which show a difference between treatments, and those which have a big random effect. And, of course, those with both. Once we have a list of these, we can ask whether particular types of gene are behaving in interesting ways, or whether it seems random.
A randomly selected image of a lot of spots
Now, the traditional way of dealing with this is to calculate p-values, and declare the genes with p<0.05 (possibly after a correction for multiple tests) interesting. For reason why that's a bad idea go here, and don’t come back until you’ve fully digested the lesson, and have taken to heart that the author is the greatest thinker since the inventor of the number 54. What makes this worse is the random effect: the test would be of whether the variance was greater than zero. But in reality the variance is always >0, so the test is really really silly.
Instead we could simply set a threshold, say a value of 2, and say any gene with a treatment effect larger than this (or smaller than -2) is “significant”. But if we do that, we will tend to pick genes with less reliable estimates (because they are more likely by chance to get beyond the threshold).
So, having eliminated the two obvious approaches, what to do? At the moment I don’t know, which is why I’m blogging this: I’m hoping for some suggestions, or at least hints. I’m particularly vague on what will be done with the genes afterwards. It seems that people produce summaries like pie charts, and say things like “this group of genes is over-represented”. What is not clear to me is what else is done: what questions are being asked?
If the questions are clear and precise, then I think the statistics can take over: for example we can weight the importance of genes by the reliability of the estimates (e.g. through their standard errors). It might even be unnecessary to pick out important genes: we can use all of them, or be liberal in picking out genes.
So, I’m interested to hear any thoughts on this. In particular, if we have a treatment, and some genes that it (might) affect, what sort of questions are being asked about those genes? How do we go from this list of genes to something that’s useful? Or, better, how do we want go from this list of genes to something that’s useful? If we can refine the biological questions, we can wheel out the statistical machinery more effectively.
As you can see, this is a research problem, so any useful ideas might turn into a paper. Contributions will be fully acknowledged, of course.
You can always manually check all the genes in the pathways/processes you are interested in for their values and see which ones give basically the right kind of signal (upregulated, downregulated). Make your own criteria. Be specific in the sense that you apply your knowledge of the literature and of the system the microarray data is derived from. Microarray is merely a tool after all to get insight into a process.
That only works if you have no intention on publishing the actual microarray data itself.
You would have to validate the information anyway with other (more reliable) techniques. And then you might actually still have to do some proper experiments.
Or at least that is often the case if you are planning on publishing somewhere decent.
In conclusion, I suggest the application of the educated guess as a selection method.
I don’t know how this would work here, but sometimes you can rank them. So instead of just saying the treshold is 2, and 1.9 is not a hit, you can rank everything, and it might go something like this: 2.5, 2.5, 2.4, 2.4, 2.3, 2.2, 2.1, 2.1, 2.0, 1.9, 1.9, 1.8, 0.3, 0.3, 0.2, 0. (Dramatization!) So clearly there is a huge drop somewhere, and that is probably the cutoff point.
But not all screens have these obvious drops, so your mileage may vary.
And what Mark said: whatever cutoff point you pick, validate above and below to make sure it’s right.
I was wondering through the Met one day and came across this picture by Paul Klee.
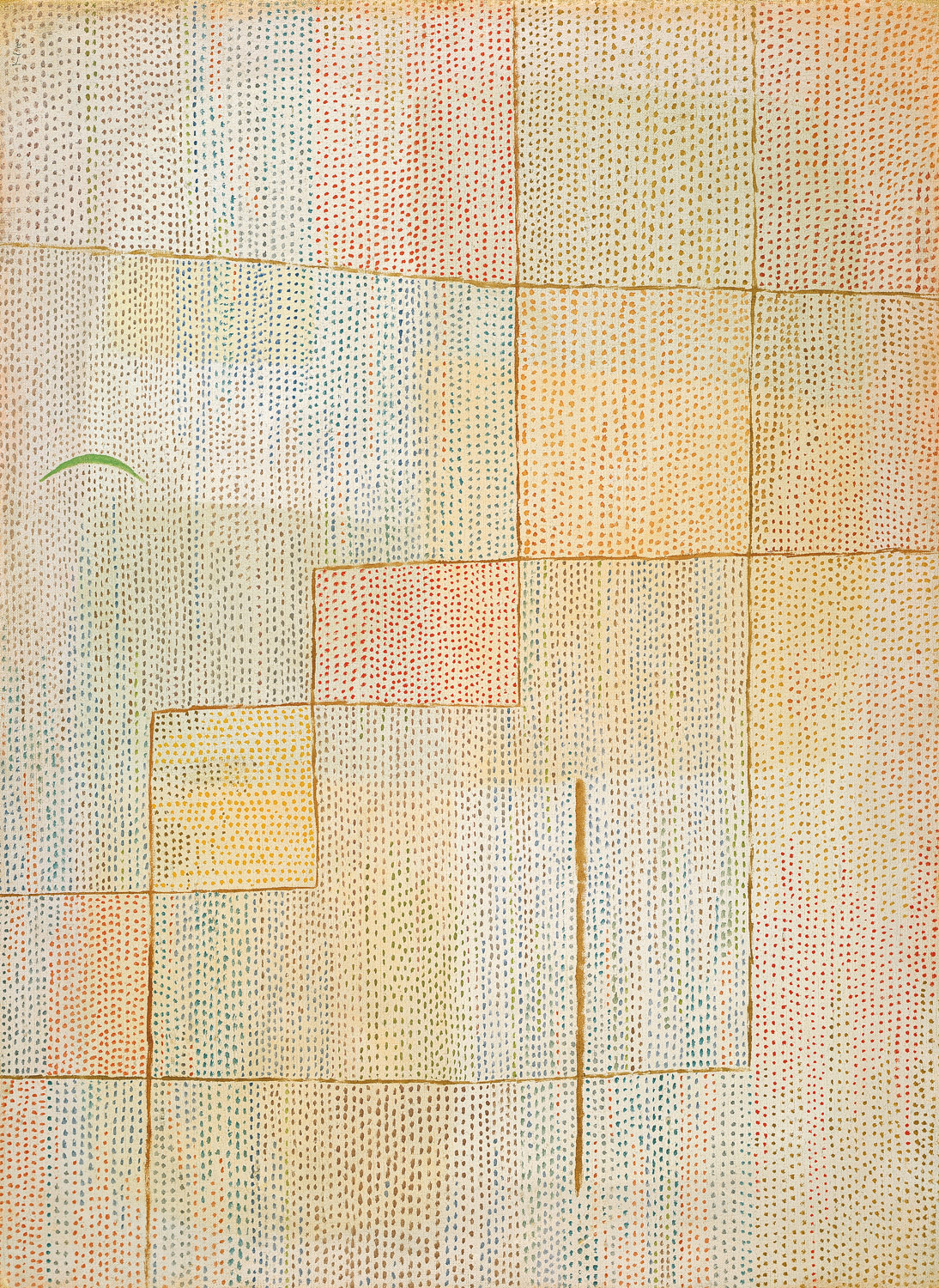
People around me were wondering why I started laughing. Maybe you’ll get the joke too when you see the title… Clarification.
Sorry Bob, I realise that’s not much help.
@ Mark – alas we’re not looking at a specific pathway (and not in a model organism either). I like the idea of reducing the problem down to pathways, though. Wasn’t someone in Viikki working on that? We’re not really sure what the treatment will affect.
@ Eva – there aren’t any obvious drops, alas. I’m prefer to avoid using cut-offs, or make the cut-off low enough that anything left out won’t have any effect. I’m hoping I can use some form of weighting of the effects so that everything will work. But I’d need to have a clearer idea about what we want to do, so I can work out how to do it.
@ Darren – it may not have helped, but I love it!
My approach to this kind of problem (not knowing the experimental details here): I would find a strategy that reduces the number of candidate genes to a reasonable number, e.g. not more than at most than 50 genes. These genes can then be validated with Northern Blots or quantitative RT-PCR, and their putative or known biological function can be looked up in the literature to select the most interesting 1-3 genes.
To get to these ≤ 50 genes, I would first set a reasonable threshhold (e.g. 2fold difference in expression). Now I only pick those genes that fulfill these threshhold conditions in multiple different experiments, where the difference could be different timepoints, similar cell lines or similar treatment conditions (or whatever makes sense in your experiment).
This strategy is obviously intended for using the microarray analysis as a screen to identify interesting candidate genes. The strategy would be different if you were interested in describing the global expresion pattern of your experimental conditions.
Bob> if you don’t want to look at “which genes are changing more than 2 up or down” in order to make a list of genes that changes, the pathway/genes in a pathway would be an interesting one. That way you can also define the question much more – depending on what kind of treatment you are doing etc.
That selection of course makes it a smaller group, defined by you so you might miss out on something else. [which is one thing why I think people tend to do “overall searches”… fear of missing things.] However, this in itself might be a good thing – that you have a defined group of genes that you want to study in the light of these treatments and might give you more defined answers.
For example, you might know that these treatments will be involved in inflammatory responses?! Then look at genes involved in these and see if some conclusions can be drawn from that.
Another option might be to first compare the sets all over on “up and down” regulated genes and see if you find some things in that. All up regulated genes in treatment A are down regulated in treatment B etc. Then you can look more closely to those subsets?!
Just throwing out ideas…. since I am not a human geneticist 😉
Getting back to pathways, if that appeals to you (and it should, because they make biological sense – no protein product of a transcript exists in a vacuum, and the cell is rife with feedback loops) I’d second the recommendation of Ingenuity as mentioned on FriendFeed, or the open more-or-less equivalent Gene Ontology and KEGG pathways.
But don’t throw away thresholds entirely. Though many components of a pathway are not going to vary substantially so as to be “visible”, at least one or two ought to. Can’t you use a sort of recursion to lower the water level, as it were, and see which rocks poke their heads out first, then next, and further down? And you can group those into functional pools to some extent – GO is not the be-all and end-all, of course, but it’s a start.
Overall, I do rather subscribe to mark’s view – microarrays are not an end in themselves, nor enough to prove a hypothesis. Like binocular vision, you need at least one other validating and consistent technical approach to believe what you see. When you say you’re not sure what the treatment will affect, there must be some obvious first candidates in its initial metabolism, at least?
By the way, there is this recent review – perhaps this “modular” approach is applicable to your situation?
I’ve been digging into network theory lately, so this idea comes immediately to mind: overlay what we know of the gene activation network(s) over the data in the array, and calculate a score for each gene based on how it relates to nearby genes (in the network). You could look for all sorts of different relationships: genes that are part of a close neighborhood that all tend to respond to the treatment, genes that stand out in their response relative to their close neighborhood, etc.
I suspect that you could substantially reduce the number of data points requiring individual inspection by this method, even though we’re only beginning to establish the totality of network relationships. Of course, groups of genes that respond together but aren’t in a close neighborhood according to current data are candidates for research to see if there are activation links.